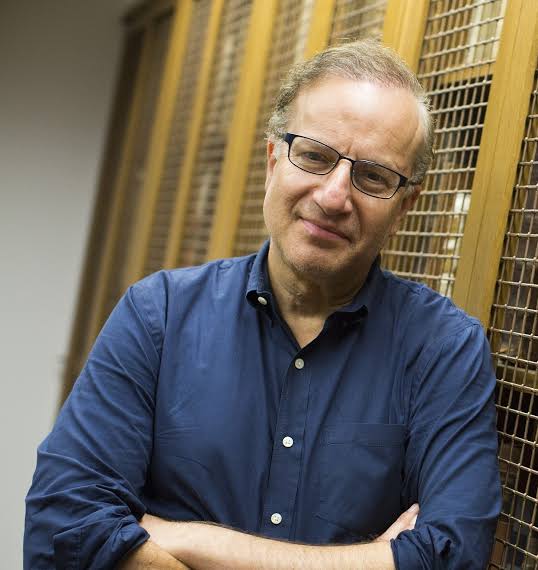
Generalizability in Machine Learning for Health: Critical for Robustness, or a Distraction from Specific Validation?
Bio: Isaac “Zak” Kohane, MD, PhD, is the inaugural chair of Harvard Medical School’s Department of Biomedical Informatics, whose mission is to develop the methods, tools, and infrastructure required for a new generation of scientists and care providers to move biomedicine rapidly forward by taking advantage of the insight and precision offered by big data. Kohane develops and applies computational techniques to address disease at multiple scales, from whole health care systems to the functional genomics of neurodevelopment. He also has worked on AI applications in medicine since the 1990’s, including automated ventilator control, pediatric growth monitoring, detection of domestic abuse, diagnosing autism from multimodal data and most recently assisting clinicians using whole genome sequence and clinical histories to diagnose rare or unknown disease patients. His most urgent question is how to enable doctors to be most effective and enjoy their profession when they enter into a substantial symbiosis with machine intelligence. He is a member of the National Academy of Medicine, the American Society for Clinical Investigation and the American College of Medical Informatics.
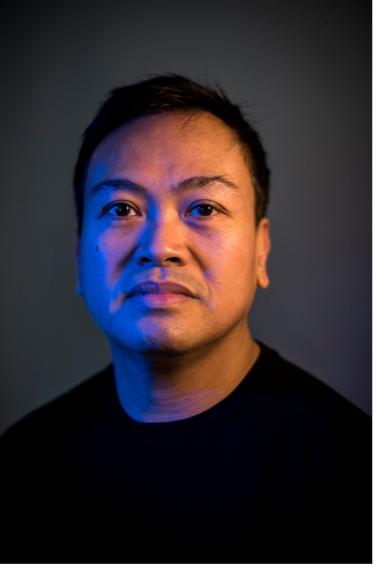
Generalizability in Machine Learning for Health: Critical for Robustness, or a Distraction from Specific Validation?
Bio: Leo focuses on scaling clinical research to be more inclusive through open access data and software, particularly for limited resource settings; identifying bias in the data to prevent them from being encrypted in models and algorithms; and redesigning research using the principles of team science and the hive learning strategy.
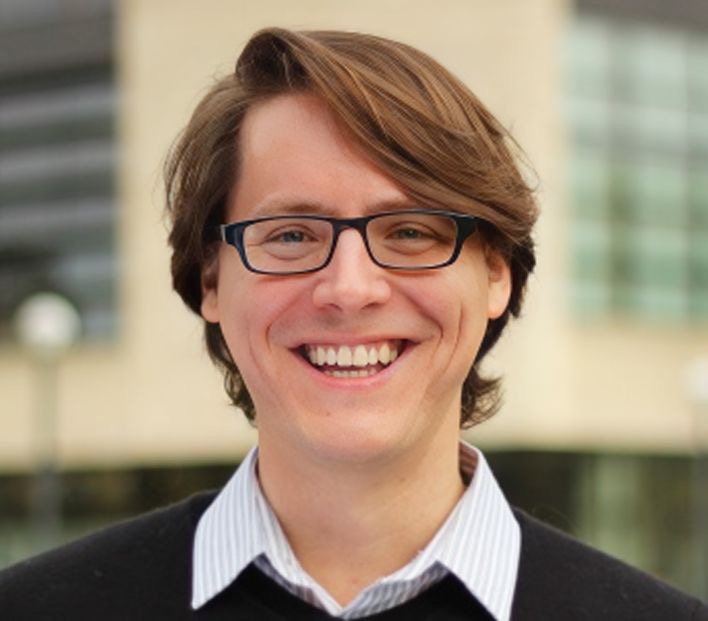
Generalizability in Machine Learning for Health: Critical for Robustness, or a Distraction from Specific Validation?
Bio: Jason Fries is a research scientist at the Shah Lab at Stanford University. His work is centered on enabling domain experts to easily construct and modify machine learning models, particularly in the field of medicine where expert-labeled training data are hard to acquire. His research interests include weakly supervised machine learning, foundation models for medicine, and data-centric AI.
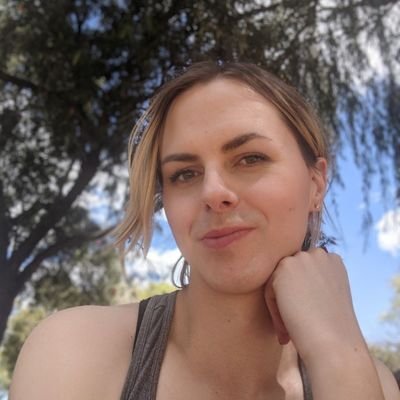
Generalizability in Machine Learning for Health: Critical for Robustness, or a Distraction from Specific Validation?
Bio: Lauren Oakden-Rayner is a radiologist and Senior Research Fellow at the Australian Institute for Machine Learning, University of Adelaide. Her research primarily focuses on medical AI safety, specifically addressing the issues of model robustness, generalization, evaluation, and fairness. Lauren is also involved in supervising students and working on various medical AI projects, reviewing MOOCs on her blog, and advocating for diversity in her group and Institute.
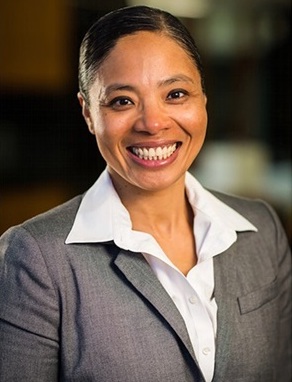
Generalizability in Machine Learning for Health: Critical for Robustness, or a Distraction from Specific Validation?
Bio: Maia Hightower, MD, MBA, MPH, is an accomplished healthcare IT executive and internist. She currently serves as the executive Vice President and Chief Digital & Technology Officer at the University of Chicago Medicine and the CEO and co-founder of Equality AI, a startup aimed at achieving health equity through responsible AI and machine-learning operations. Previously, she was the chief medical information officer and associate chief medical officer at University of Utah Health and served in similar roles at University of Iowa Health Care and Stanford Health Care. Dr. Hightower's work has focused on leveraging digital technology to address health inequities and promoting diversity and inclusion within healthcare IT systems. Her leadership in the field has earned her widespread recognition.
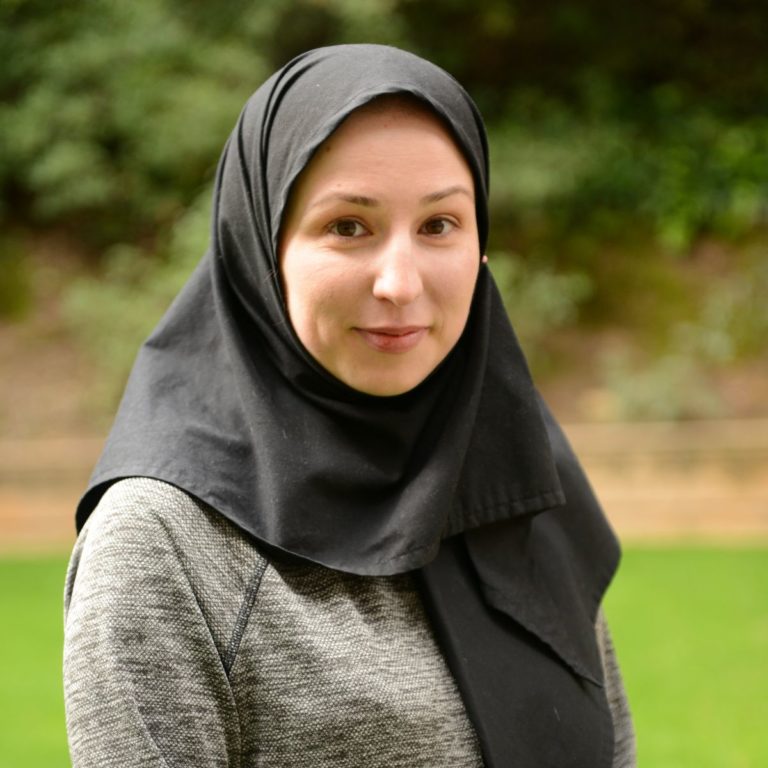
Sharing Health Data in an Age of Generative AI: Risks, Limitations, and Solutions
Bio: Marzyeh Ghassemi is an assistant professor and the Hermann L. F. von Helmholtz Professor with appointments in the Department of Electrical Engineering and Computer Science and the Institute for Medical Engineering & Science at MIT. Ghassemi’s research interests span representation learning, behavioral ML, healthcare ML, and healthy ML. One of her focuses is on real-world applications of machine learning, such as turning diverse clinical data into cohesive information with the ability to predict patient needs. Ghassemi has received BS degrees in computer science and electrical engineering from New Mexico State University, an MSc degree in biomedical engineering from Oxford University, and PhD in computer science from MIT.
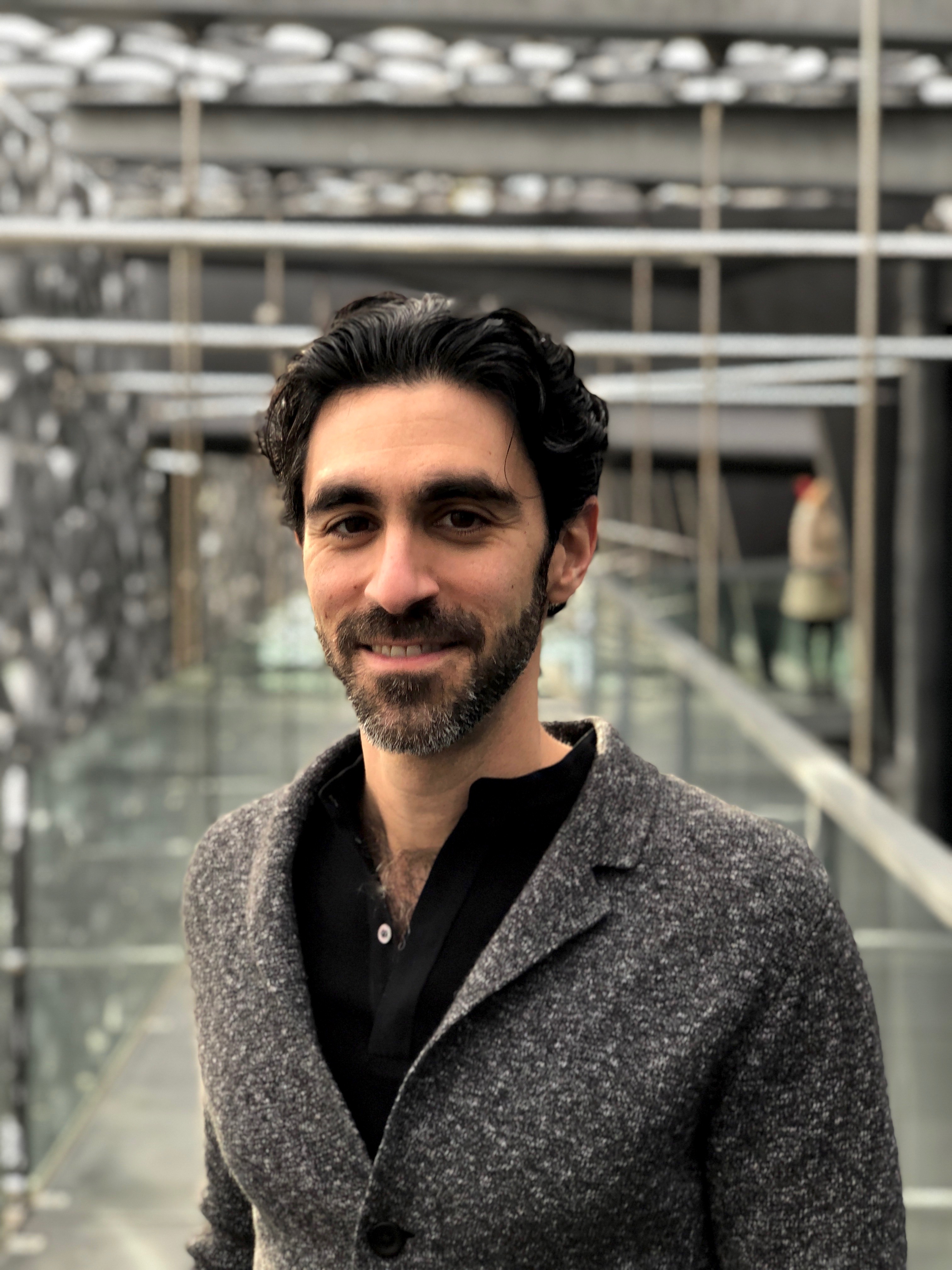
Sharing Health Data in an Age of Generative AI: Risks, Limitations, and Solutions
Bio: Ziad Obermeyer is Associate Professor and Blue Cross of California Distinguished Professor at UC Berkeley, where he works at the intersection of machine learning and health. He is a Chan Zuckerberg Biohub Investigator, a Faculty Research Fellow at the National Bureau of Economic Research, and was named an Emerging Leader by the National Academy of Medicine. Previously, he was Assistant Professor at Harvard Medical School, and continues to practice emergency medicine in underserved communities.
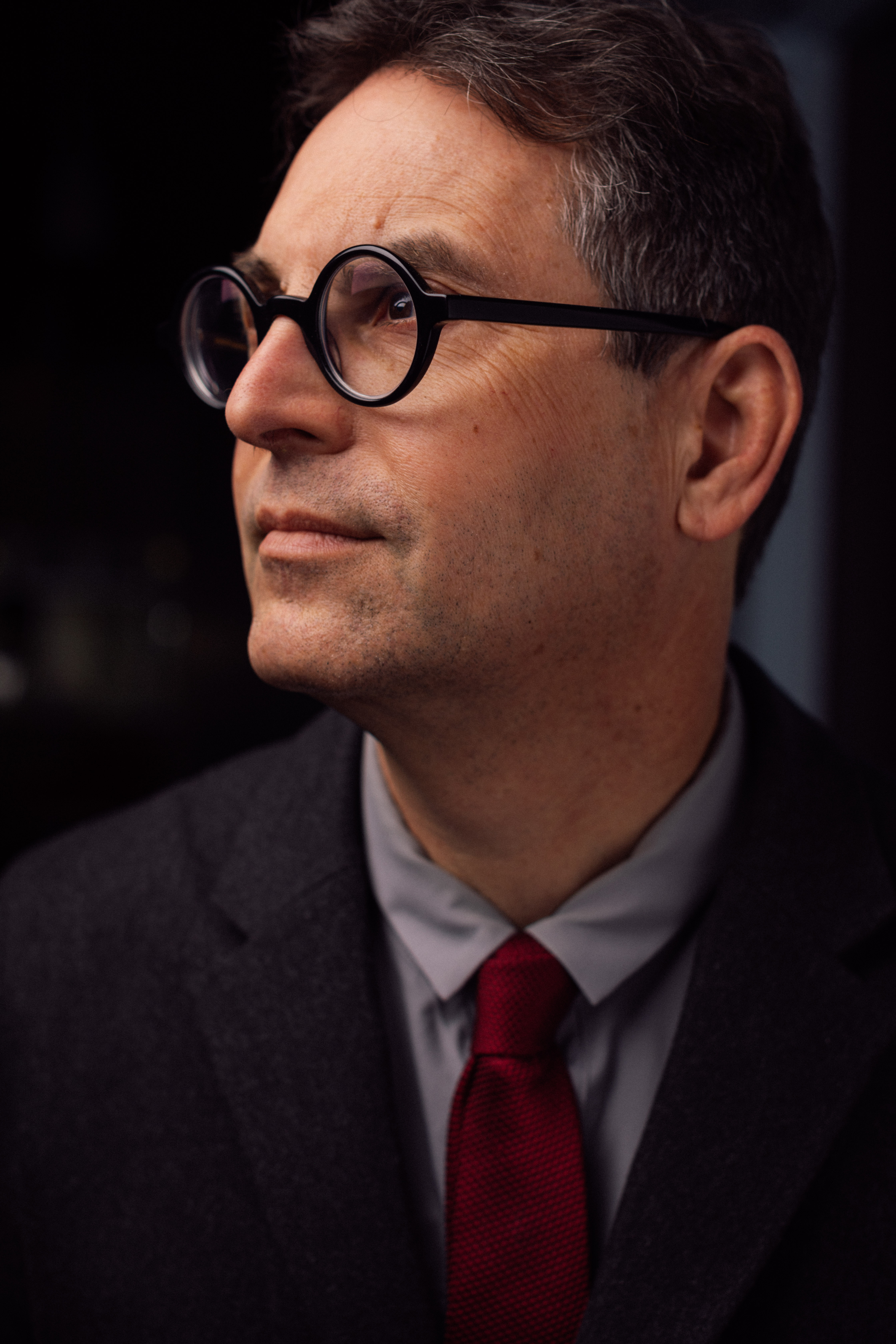
Sharing Health Data in an Age of Generative AI: Risks, Limitations, and Solutions
Bio: Dr. Halamka is an emergency medicine physician, medical informatics expert and president of the Mayo Clinic Platform, which is focused on transforming health care by leveraging artificial intelligence, connected health care devices and a network of partners. Dr. Halamka has been developing and implementing health care information strategy and policy for more than 25 years. Previously, he was executive director of the Health Technology Exploration Center for Beth Israel Lahey Health, chief information officer at Beth Israel Deaconess Medical Center, and International Healthcare Innovation Professor at Harvard Medical School. He is a member of the National Academy of Medicine.
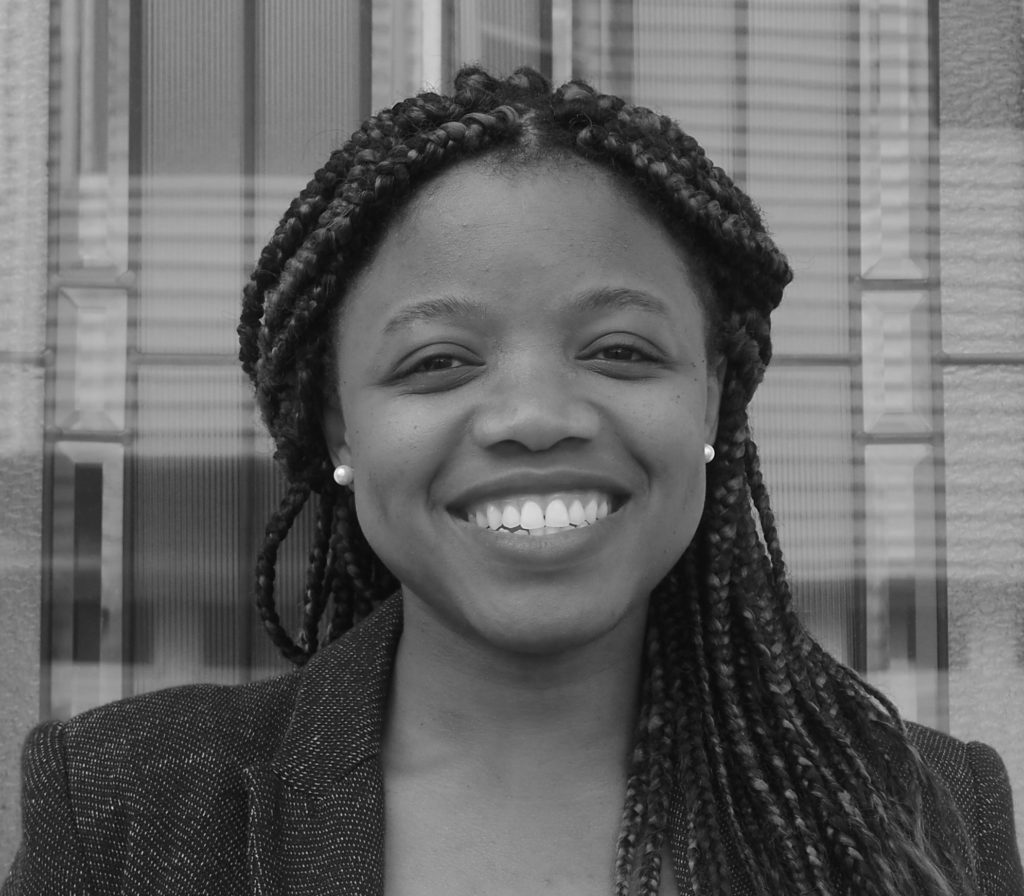
Sharing Health Data in an Age of Generative AI: Risks, Limitations, and Solutions
Bio: Elaine Nsoesie is an Associate Professor at Boston University's School of Public Health and a leading voice in the use of data and technology to advance health equity. She is leads the Racial Data Tracker project at Boston University's Center for Antiracist Research and serves as a Senior Advisor to the Artificial Intelligence/Machine Learning Consortium to Advance Health Equity and Researcher Diversity (AIM-AHEAD) program at the National Institutes of Health. Dr. Nsoesie has published extensively on the use of data from social media, search engines, and cell phones for public health surveillance and is dedicated to increasing representation of underrepresented communities in data science. She completed her PhD in Computational Epidemiology from Virginia Tech and has held postdoctoral positions at Harvard Medical School and Boston Children's Hospital.
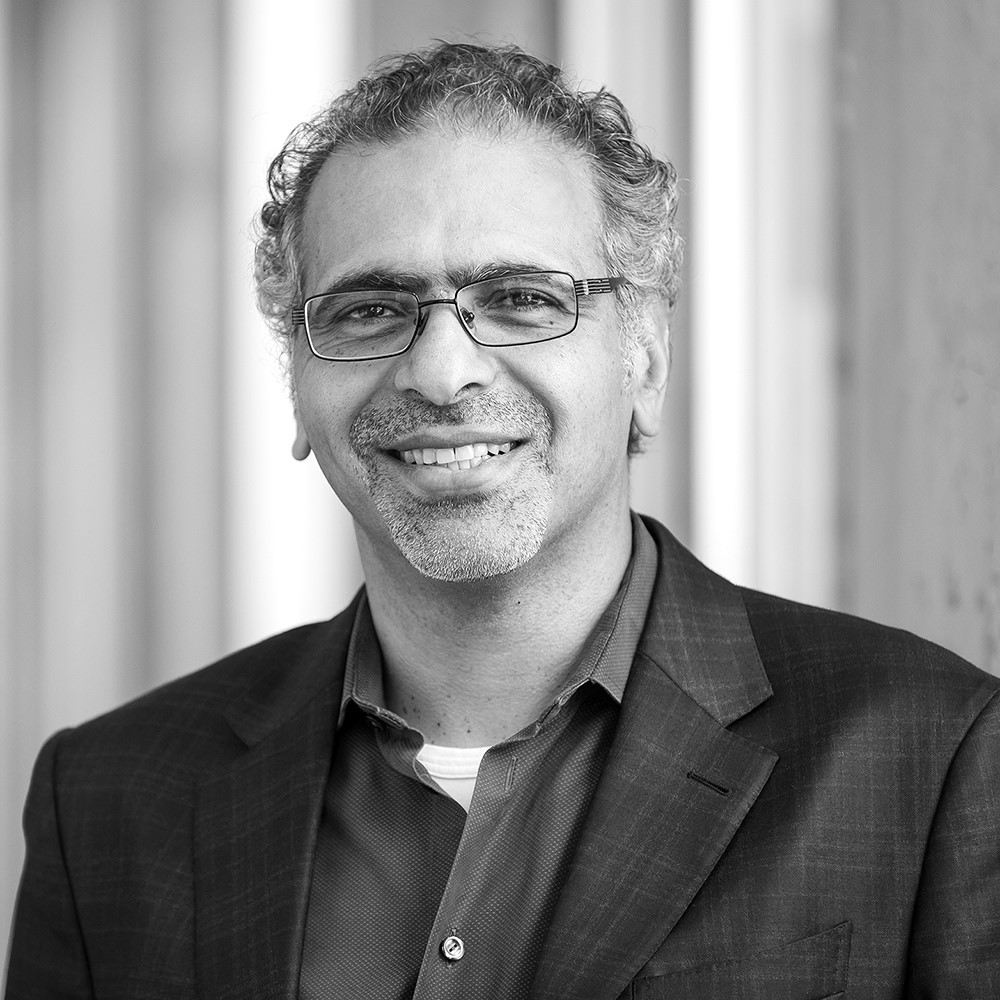
Sharing Health Data in an Age of Generative AI: Risks, Limitations, and Solutions
Bio: Dr. Khaled El Emam is the Canada Research Chair (Tier 1) in Medical AI at the University of Ottawa, where he is a Professor in the School of Epidemiology and Public Health. He is also a Senior Scientist at the Children’s Hospital of Eastern Ontario Research Institute and Director of the multi-disciplinary Electronic Health Information Laboratory, conducting research on privacy enhancing technologies to enable the sharing of health data for secondary purposes, including synthetic data generation and de-identification methods. Khaled is a co-founder of Replica Analytics, a company that develops synthetic data generation technology, which was recently acquired by Aetion. As an entrepreneur, Khaled founded or co-founded six product and services companies involved with data management and data analytics, with some having successful exits. Prior to his academic roles, he was a Senior Research Officer at the National Research Council of Canada. He also served as the head of the Quantitative Methods Group at the Fraunhofer Institute in Kaiserslautern, Germany. He participates in a number of committees, number of the European Medicines Agency Technical Anonymization Group, the Panel on Research Ethics advising on the TCPS, the Strategic Advisory Council of the Office of the Information and Privacy Commissioner of Ontario, and also is co-editor-in-chief of the JMIR AI journal. In 2003 and 2004, he was ranked as the top systems and software engineering scholar worldwide by the Journal of Systems and Software based on his research on measurement and quality evaluation and improvement. He held the Canada Research Chair in Electronic Health Information at the University of Ottawa from 2005 to 2015. Khaled has a PhD from the Department of Electrical and Electronics Engineering, King's College, at the University of London, England.
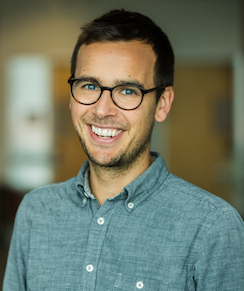
Machine Learning for Healthcare in the Era of ChatGPT
Bio: Byron Wallace is the Sy and Laurie Sternberg Interdisciplinary Associate Professor and Director of the BS in Data Science program at Northeastern University in the Khoury College of Computer Sciences. His research is primarily in natural language processing (NLP) methods, with an emphasis on their application in healthcare and the challenges inherent to this domain.
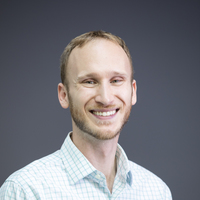
Machine Learning for Healthcare in the Era of ChatGPT
Bio: Tristan Naumann is a Principal Researcher in Microsoft Research’s Health Futures working on problems related to clinical and biomedical natural language processing (NLP). His research focuses on exploring relationships in complex, unstructured healthcare data using natural language processing and unsupervised learning techniques. He is currently serving as General Chair of NeurIPS and co-organizer of the Clinical NLP workshop at ACL. Previously, he has served as General Chair and Program Chair of the AHLI Conference on Health, Inference, and Learning (CHIL) and Machine Learning for Health (ML4H). His work has appeared in KDD, AAAI, AMIA, JMIR, MLHC, ACM HEALTH, Cell Patterns, Science Translational Medicine, and Nature Translational Psychiatry.
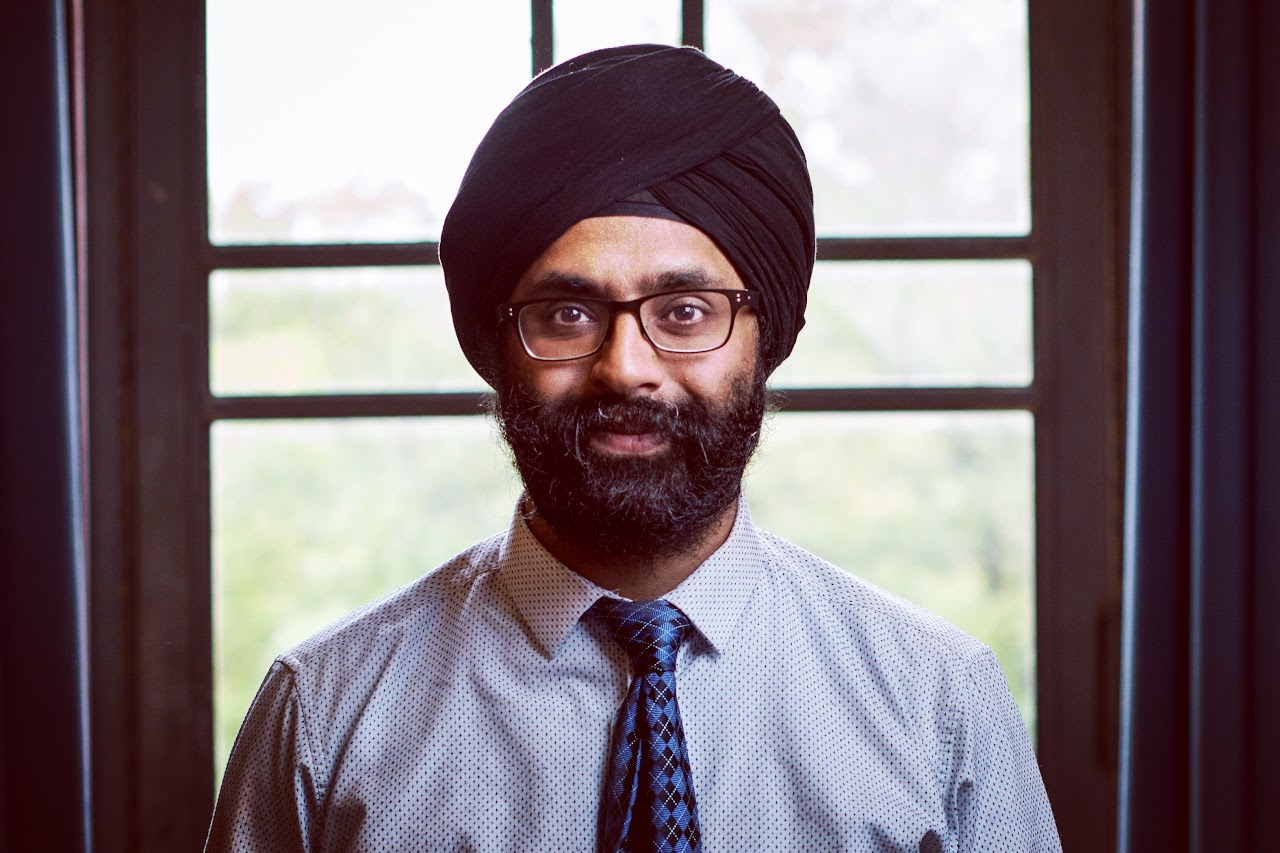
Machine Learning for Healthcare in the Era of ChatGPT
Bio: Karandeep Singh, MD, MMSc, is an Assistant Professor of Learning Health Sciences, Internal Medicine, Urology, and Information at the University of Michigan. He directs the Machine Learning for Learning Health Systems (ML4LHS) Lab, which focuses on translational issues related to the implementation of machine learning (ML) models within health systems. He serves as an Associate Chief Medical Information Officer for Artificial Intelligence for Michigan Medicine and is the Associate Director for Implementation for U-M Precision Health, a Presidential Initiative focused on bringing research discoveries to the bedside, with a focus on prediction models and genomics data. He chairs the Michigan Medicine Clinical Intelligence Committee, which oversees the governance of machine learning models across the health system. He teaches a health data science course for graduate and doctoral students, and provides clinical care for people with kidney disease. He completed his internal medicine residency at UCLA Medical Center, where he served as chief resident, and a nephrology fellowship in the combined Brigham and Women’s Hospital/Massachusetts General Hospital program in Boston, MA. He completed his medical education at the University of Michigan Medical School and holds a master’s degree in medical sciences in Biomedical Informatics from Harvard Medical School. He is board certified in internal medicine, nephrology, and clinical informatics.
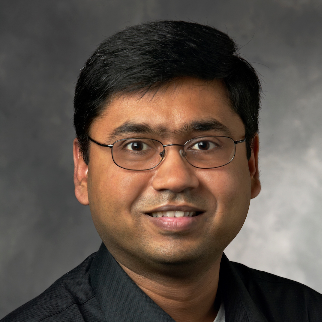
Machine Learning for Healthcare in the Era of ChatGPT
Bio: Dr. Nigam Shah is Professor of Medicine at Stanford University, and Chief Data Scientist for Stanford Health Care. His research group analyzes multiple types of health data (EHR, Claims, Wearables, Weblogs, and Patient blogs), to answer clinical questions, generate insights, and build predictive models for the learning health system. At Stanford Healthcare, he leads artificial intelligence and data science efforts for advancing the scientific understanding of disease, improving the practice of clinical medicine and orchestrating the delivery of health care. Dr. Shah is an inventor on eight patents and patent applications, has authored over 200 scientific publications and has co-founded three companies. Dr. Shah was elected into the American College of Medical Informatics (ACMI) in 2015 and was inducted into the American Society for Clinical Investigation (ASCI) in 2016. He holds an MBBS from Baroda Medical College, India, a PhD from Penn State University and completed postdoctoral training at Stanford University.
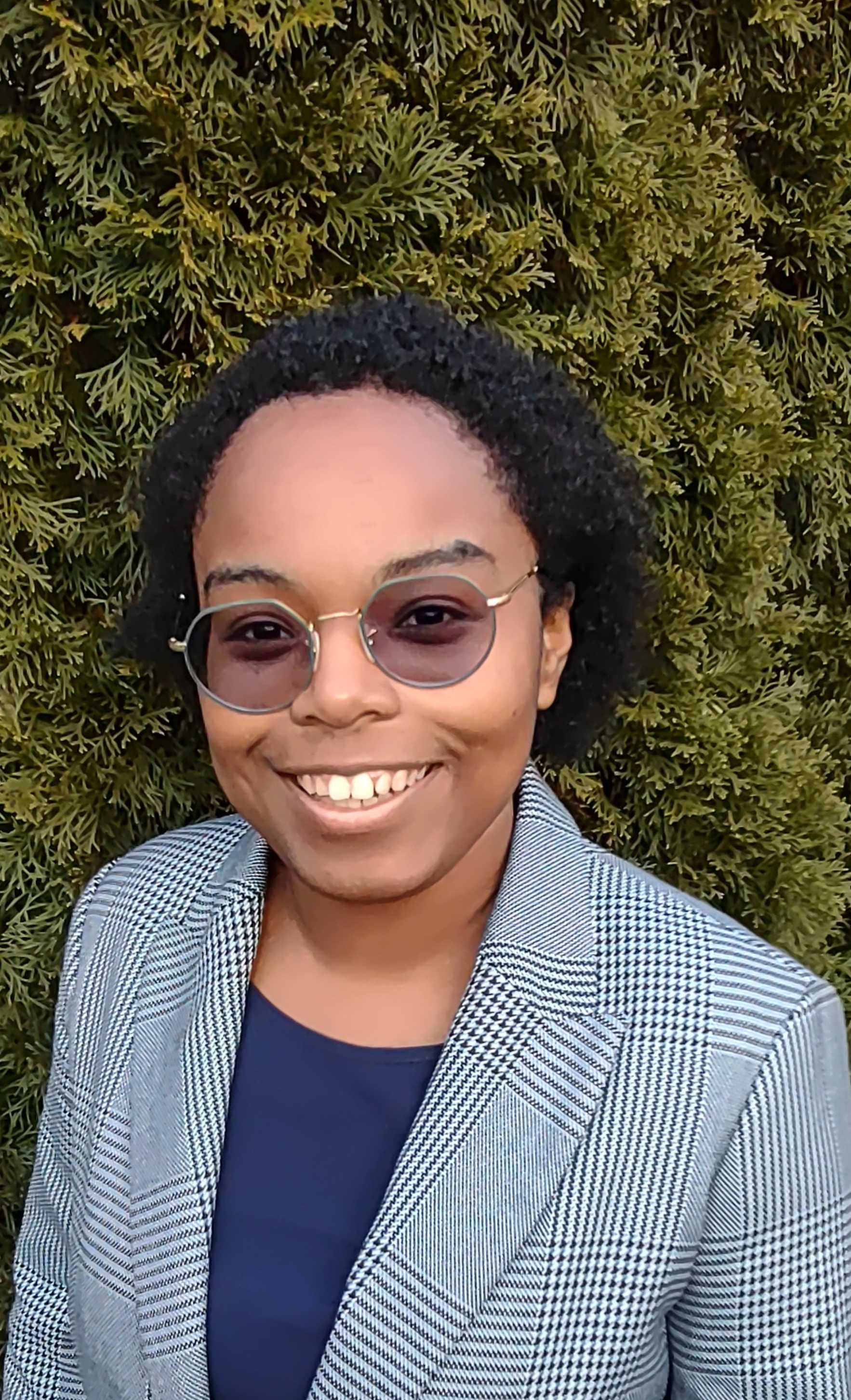
Machine Learning for Healthcare in the Era of ChatGPT
Bio: Saadia Gabriel is currently a MIT CSAIL Postdoctoral Fellow. She is also an incoming NYU Faculty Fellow and will start as an Assistant Professor at UCLA in 2024. She completed her PhD at the University of Washington, where she was advised by Prof. Yejin Choi and Prof. Franziska Roesner. Her research revolves around natural language processing and machine learning, with a particular focus on building systems for understanding how social commonsense manifests in text (i.e. how do people typically behave in social scenarios), as well as mitigating spread of false or harmful text (e.g. Covid-19 misinformation). Her work has been covered by a wide range of media outlets like Forbes and TechCrunch. It has also received a 2019 ACL best short paper nomination, a 2019 IROS RoboCup best paper nomination and won a best paper award at the 2020 WeCNLP summit.