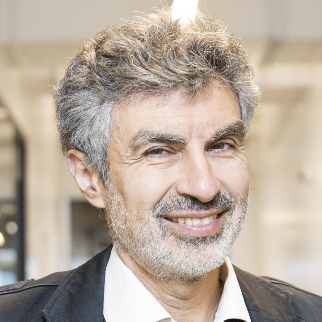
Machine Learning Challenges in the Fight for Social Good - the Covid-19 Case
Abstract: This talk outlines two Mila projects aimed at fighting the Covid-19 pandemic which are part of Mila's AI for Humanity mission. The first one is about discovering antivirals, either via repurposing several existing drugs using graph neural networks or via discovering new drug-like molecules using reinforcement learning and docking simulations to search in the molecular space. The second project is about using machine learning to provide early warning signals to people who are contagious -- especially if they don't realize that they are -- by exchanging information between phones of users who have had dangerous contacts with each other. This extends digital contact tracing by incorporating information about symptoms, medical condition and behavior (like wearing a mask) and relies on a sophisticated epidemiological model at the individual levels in which we can simulate different individual-level and society-level strategies.
Bio: Yoshua Bengio is Professor in the Computer Science and Operations Research departments at U. Montreal, founder and scientific director of Mila and of IVADO. He is a Fellow of the Royal Society of London and of the Royal Society of Canada, has received a Canada Research Chair and a Canada CIFAR AI Chair and is a recipient of the 2018 Turing Award for pioneering deep learning, is an officer of the Order of Canada, a member of the NeurIPS advisory board, co-founder and member of the board of the ICLR conference, and program director of the CIFAR program on Learning in Machines and Brains. His goal is to contribute to uncovering the principles giving rise to intelligence through learning, as well as favour the development of AI for the benefit of all.
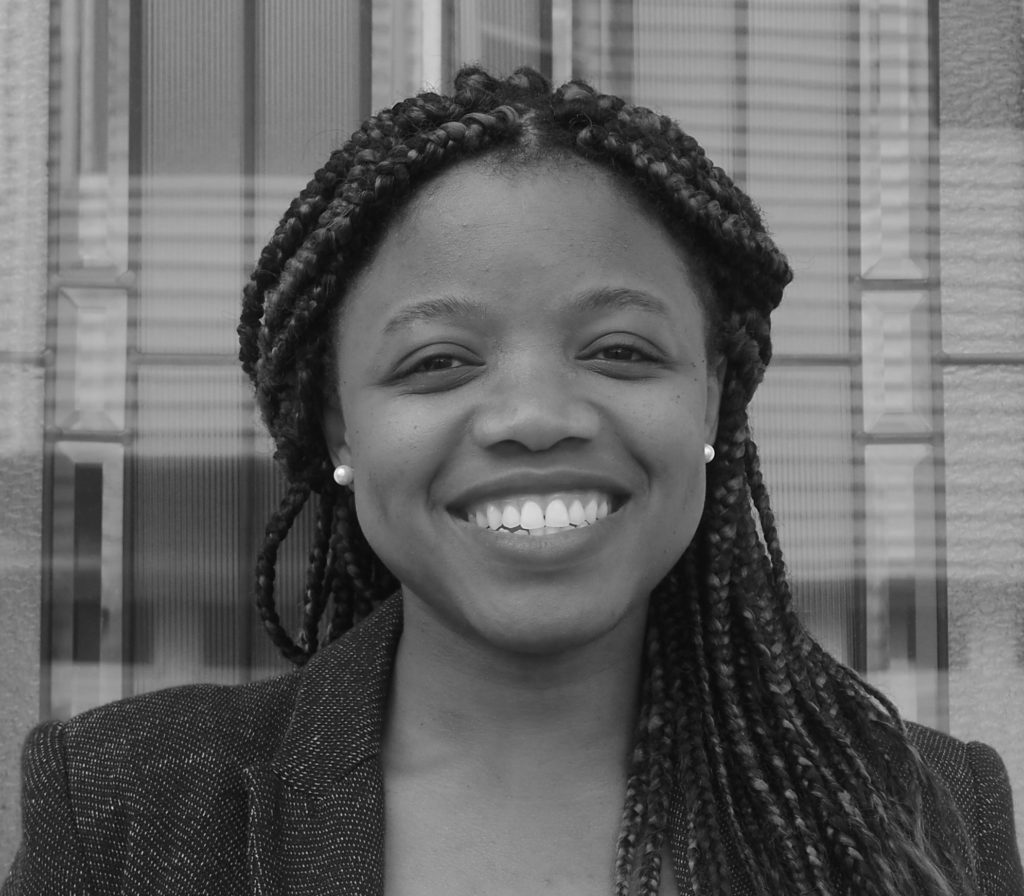
Digital Platforms & Public Health in Africa
Abstract: Countries in Sub-Saharan Africa are facing a double burden of infectious and noncommunicable diseases. The burden of noncommunicable diseases such as, diabetes and hypertension, are expected to continue increasing. Digital data and tools that can be used to study the patterns of health and disease in populations offer opportunities for improving public health. Digital platforms such as, social media, search engines, and internet forums, have been widely accepted in Sub-Saharan Africa for health information seeking and sharing. These tools can be used to improve public health in Sub-Saharan Africa in three ways: (1) monitoring health information seeking and providing health education, (2) monitoring risk factors, and (3) monitoring disease incidence. However, in order for these tools to be effective, it is important to consider and incorporate into analytical processes the distinct social, cultural, and economic context in Sub-Saharan African countries.
Bio: Dr. Nsoesie is an Assistant Professor of Global Health at Boston University (BU) School of Public Health. She is also a BU Data Science Faculty Fellow as part of the BU Data Science Initiative at the Hariri Institute for Computing and a Data and Innovation Fellow at The Directorate of Science, Technology and Innovation (DSTI) in the Office of the President in Sierra Leone. Dr. Nsoesie applies data science methodologies to global health problems, using digital data and technology to improve health, particularly in the realm of surveillance of chronic and infectious diseases. She has worked with local public health departments in the United States and international organizations. She completed her postdoctoral studies at Harvard Medical School, and her PhD in Computational Epidemiology from the Genetics, Bioinformatics and Computational Biology program at Virginia Tech. She also has an MS in Statistics and a BS in Mathematics. She is the founder of Rethé – an initiative focused on providing scientific writing tools and resources to student communities in Africa in order to increase representation in scientific publications. She has written for NPR, The Conversation, Public Health Post and Quartz. Dr. Nsoesie was born and raised in Cameroon.
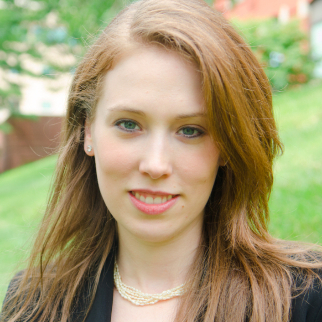
Machine Learning in Health Care: Too Important to Be a Toy Example
Abstract: The massive size of the health care sector make data science applications in this space particularly salient for social policy. An overarching theme of this keynote is that developing machine learning methodology tailored to specific substantive health problems and the associated electronic health data is critical given the stakes involved, rather than eschewing complexity in simplified scenarios that may no longer represent an actual real-world problem.
Bio: Sherri Rose, Ph.D. is an Associate Professor of Health Care Policy at Harvard Medical School and Co-Director of the Health Policy Data Science Lab. Her research in health policy focuses on risk adjustment, comparative effectiveness, and health program evaluation. Dr. Rose coauthored the first book on machine learning for causal inference and has published work across fields, including in Biometrics, JASA, PMLR,Journal of Health Economics, and NEJM. She currently serves as co-editor of the journal Biostatistics and is Chair-Elect of the American Statistical Association’s Biometrics Section. Her honors include the ISPOR Bernie J. O’Brien New Investigator Award for exceptional early career work in health economics and outcomes research and an NIH Director’s New Innovator Award to develop machine learning estimators for generalizability in health policy.
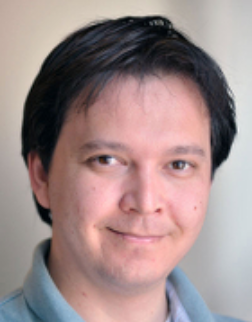
Incorporating Domain Knowledge into Deep Learning Models
Abstract: Details to be confirmed.
Bio: Dr. Ruslan Salakhutdinov is a UPMC professor of Computer Science at Carnegie Mellon University. He has served as an area chair for NIPS, ICML, CVPR, and ICLR. He holds a PhD from University of Toronto and completed postdoctoral training at Massachusetts Institute of Technology.
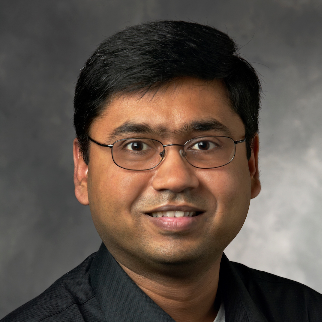
A framework for shaping the future of AI in healthcare
Abstract: In this session we will explore strategies for, and issues involved in, bringing Artificial Intelligence (AI) technologies to the clinic, safely and ethically. We will discuss the characteristics of a sound data strategy for powering a machine learning (ML) health system. The session introduces a frame-work for analyzing the utility of ML models in healthcare and discusses the implicit assumptions in aligning incentives for AI guided healthcare actions.
Bio: Dr. Nigam Shah is Associate Professor of Medicine (Biomedical Informatics) at Stanford University, and serves as the Associate CIO for Data Science for Stanford Health Care. Dr. Shah’s research focuses on combining machine learning and prior knowledge in medical ontologies to enable the learning health system. Dr. Shah was elected into the American College of Medical Informatics (ACMI) in 2015 and is inducted into the American Society for Clinical Investigation (ASCI) in 2016. He holds an MBBS from Baroda Medical College, India, a PhD from Penn State University and completed postdoctoral training at Stanford University.